In the realm of statistical analysis, high p value low chi squared Stata often sparks curiosity among researchers and students alike. This combination is frequently encountered in hypothesis testing, especially when assessing the fit of observed data against expected outcomes. For those analyzing data in Stata, understanding this interplay is critical to interpreting results accurately. This article will explore what it means to have a high p-value and a low Chi-squared value, why it matters, and how you can interpret these results in your research.
What Are high p value low chi squared Stata?
To understand the relationship between high p value low chi squared Stata, we must first define these terms. A p-value is a probability metric used to determine the significance of your test results. It helps answer the question: Are the observed differences due to random chance or a significant underlying factor? In contrast, the Chi-squared value measures the difference between observed and expected frequencies in categorical data. A low Chi-squared value indicates minimal deviation, suggesting that your observed data closely aligns with expectations.
When these two metrics are combined in tests like the Chi-squared goodness-of-fit or independence test, they provide a robust framework for evaluating hypotheses. In Stata, these tests are simple to execute but require careful interpretation to draw meaningful conclusions.
Significance of High P-Value in Statistical Testing
A high p-value, typically greater than 0.05, suggests weak evidence against the null hypothesis. This means that you fail to reject the null hypothesis, implying that the observed data could reasonably occur under it. For example, in a Chi-squared test, a high p-value indicates that the differences between observed and expected data are not statistically significant.
In practical terms, a high p-value in Stata might arise when comparing survey responses, demographic distributions, or experimental results where the observed data aligns well with theoretical models. It does not necessarily mean that the variables have no relationship; rather, the relationship is not strong enough to be statistically significant.
Understanding Low Chi-Squared Values
The Chi-squared value quantifies the extent of deviation between observed and expected data. A low Chi-squared value implies that the discrepancies between these datasets are small. This outcome often corresponds to a high p-value, as minimal differences lead to a lack of statistical significance.
For instance, in Stata, a low Chi-squared value in a goodness-of-fit test would indicate that the observed data distribution closely matches the expected one. This result is desirable when verifying the validity of theoretical models or assessing fit in categorical data.
Also Read: V4Holt
High P Value Low Chi Squared Stata: A Closer Look
The combination of High P Value Low Chi Squared Stata value is a hallmark of data consistency with the null hypothesis. In Stata, this result often occurs when running Chi-squared tests, such as the ‘tabulate’ command with the ‘chi2’ option. For example:
. tabulate variable1 variable2, chi2
This command generates a Chi-squared test for independence, reporting both the Chi-squared statistic and the p-value. A low Chi-squared value and high p-value in this output suggest that the two variables are independent.
Let’s consider a scenario: A researcher is examining whether gender influences preferences for different types of beverages. If the data shows minimal difference between observed and expected preferences, the Chi-squared value will be low, and the p-value high. This means that the null hypothesis (no association between gender and beverage preference) cannot be rejected.
Applications of High P-Value and Low Chi-Squared in Real-World Research
Understanding high p value low chi squared stata is essential for applications across various fields:
- Market Research: When analyzing customer preferences, researchers often compare observed purchase behavior with expected trends. A high p-value and low Chi-squared value indicate that customer behavior aligns with market predictions.
- Public Health Studies: In epidemiology, Chi-squared tests are used to assess the distribution of diseases across populations. A high p-value and low Chi-squared value suggest no significant variation in disease prevalence between groups.
- Education Analysis: Researchers evaluating test score distributions among different demographics might encounter this result, indicating that performance does not significantly differ by group.
Interpreting High P Value Low Chi Squared Stata
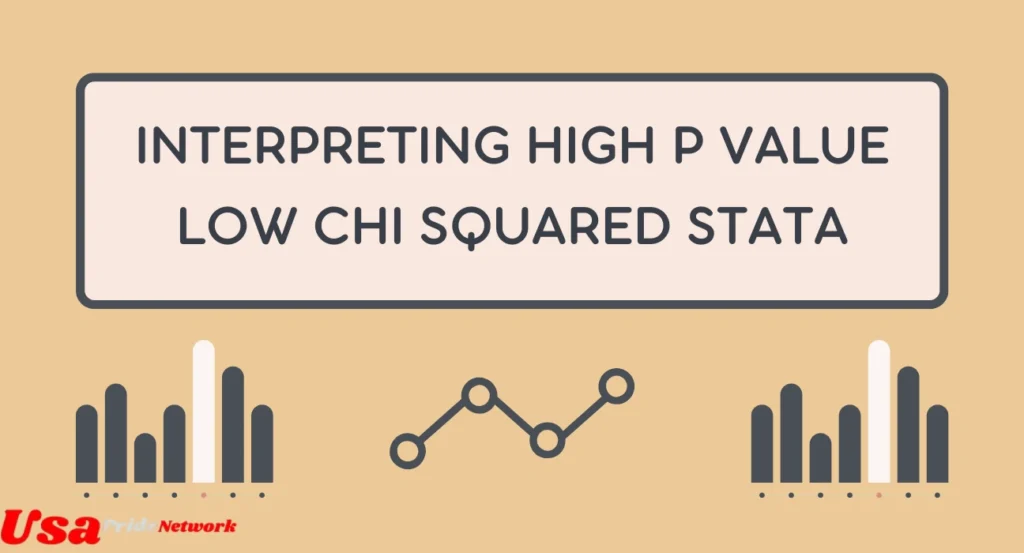
Interpreting the results of statistical tests in Stata requires careful attention to the relationship between p-value and Chi-squared value. When encountering a High P Value Low Chi Squared Stata value, consider the following:
- Context Matters: Always interpret results in the context of your research question and data characteristics.
- Sample Size: Large sample sizes can inflate Chi-squared values, potentially leading to misinterpretation. Conversely, small sample sizes might yield high p-values even when relationships exist.
- Practical Significance: A high p-value and low Chi-squared value might indicate statistical insignificance, but practical relevance should also be considered.
Example of High P-Value and Low Chi-Squared in Stata
Imagine a study examining whether a new teaching method affects students’ test scores. The researcher collects data from two groups: one using the new method and another following traditional teaching. After running a Chi-squared test in Stata, the results show:
Test Metric | Value |
Chi-Squared Value | 1.24 |
Degrees of Freedom | 2 |
P-Value | 0.68 |
The high p-value (0.68) and low Chi-squared value (1.24) suggest that the teaching methods do not significantly influence test scores. This insight helps educators decide whether to implement the new approach.
Advantages of Analyzing High P-Value and Low Chi-Squared in Stata
Stata simplifies statistical testing with user-friendly commands and outputs. The software’s ability to compute high p-value and low Chi-squared values efficiently supports researchers in:
- Validating Models: Assessing the fit of observed data to theoretical expectations.
- Testing Independence: Determining whether variables are associated or independent.
- Exploring Relationships: Investigating patterns in categorical data with precision.
Avoiding Misinterpretations
While high p value low chi squared stata results are straightforward, they can lead to misinterpretations without proper understanding. To avoid errors:
- Verify Assumptions: Ensure that Chi-squared test assumptions, such as independence of observations, are met.
- Consider Sample Size: Adjust expectations based on sample size, as it can impact p-value and Chi-squared outcomes.
- Review Data Quality: Check for errors or inconsistencies in observed and expected values.
Also Read: Fran Candelera
Advanced Insights on High P Value Low Chi Squared Stata
For advanced users, the nuances of high p value low chi squared stata outcomes can be explored further. Stata allows for combining Chi-squared results with other tests to create a comprehensive understanding of the data. For instance, incorporating logistic regression analysis or exploring residuals in the Chi-squared test can yield deeper insights. Residuals highlight the extent of discrepancy for individual cells in contingency tables, helping pinpoint areas of interest.
Moreover, Stata’s graphical capabilities can visually represent Chi-squared outcomes. Heatmaps of observed versus expected frequencies provide an intuitive understanding of data alignment. These tools make Stata invaluable for researchers handling complex datasets.
Common Pitfalls and Tips for Accurate Analysis
When dealing with high p-value and low Chi-squared results, avoid common pitfalls such as over-reliance on statistical significance without considering practical importance. Always question whether the null hypothesis’s acceptance aligns with real-world observations. Similarly, remember that a high p-value does not confirm the null hypothesis but merely indicates insufficient evidence to reject it.
For more precise results, ensure that expected frequencies meet the assumptions of the Chi-squared test. Stata’s output often flags issues like low expected counts, which can invalidate the test. In such cases, Fisher’s Exact Test or other non-parametric methods might be more appropriate.
Frequently Asked Questions
What does a High P Value Low Chi Squared Stata?
It means the observed data closely aligns with the expected data, providing weak evidence against the null hypothesis. This typically suggests no significant relationship between variables.
Why does a high p-value correspond to a low Chi-squared value in Stata?
A low Chi-squared value indicates minimal differences between observed and expected frequencies, leading to a high p-value, which implies the null hypothesis cannot be rejected.
How do you interpret high p-value and low Chi-squared results in Stata?
These results indicate that the data supports the null hypothesis, suggesting no significant association or difference between the variables being tested.
What are common scenarios for high p-value and low Chi-squared in Stata?
This outcome is common in goodness-of-fit tests, independence tests in contingency tables, and scenarios where sample data fits theoretical expectations.
Conclusion
Interpreting High P Value Low Chi Squared Stata results is a crucial skill for researchers and analysts. These outcomes provide valuable insights into data consistency and the validity of null hypotheses. By understanding the relationship between p-values and Chi-squared values, researchers can make informed decisions and draw meaningful conclusions.
Whether you are analyzing customer preferences, public health data, or educational outcomes, this knowledge empowers you to navigate statistical tests with confidence. Stata’s tools simplify the process, enabling accurate and efficient analyses. As you continue your journey in data analysis, mastering these concepts will undoubtedly enhance the depth and reliability of your research.